BEBPA Blog
Volume 2, Issue 1
Characterization of Precision and Accuracy and Total Analytical Error for Bioassays
By Laureen Little, Principal Consultant, Quality Services
We tend to think analytical validation is a fairly straight forward, almost box checking activity if a method is well-developed and suitable for its intended purpose. Indeed, validation has a long history within the pharmaceutical industry. Approximately twenty years ago (1994 and 1996), the International Council for Harmonisation (ICH) first published two guidelines for the Validation of Analytical Methods in two documents, ICH Q2A and ICH Q2B. The first document was a list of definitions and terminology, the second was a how-to guideline. These documents covered most analytical methods. The single exception was relative potency bioassays which did not have a linear dose-response curve. However, bioassay subject matter experts (SME) looked at the guidances and felt that they in fact covered most of the necessary elements. The industry happily utilized these two guidances to inform their approaches to validation for close to 20 years. These two guidances were combined in 2005 and renamed ICHQ2(R1). An updated version of this document was implemented in June 2024. This new version updates some of the terminology, clarifies the use of the guideline for methods with non-linear dose-response curves and discusses how validation fits it in with some of the other ICH Quality guidelines, such as ICH Q14.
An iconic validation performance characteristics table was published in ICH Q2B (and Q2(R1)). It described the characteristics of each type of analytical method and delineated which characteristic should be included in which validation. These four characteristics include:
- Precision (Repeatability and Intermediate)
- Accuracy
- Working Range (Suitability of calibration model, lower and upper range limit)
- Specificity
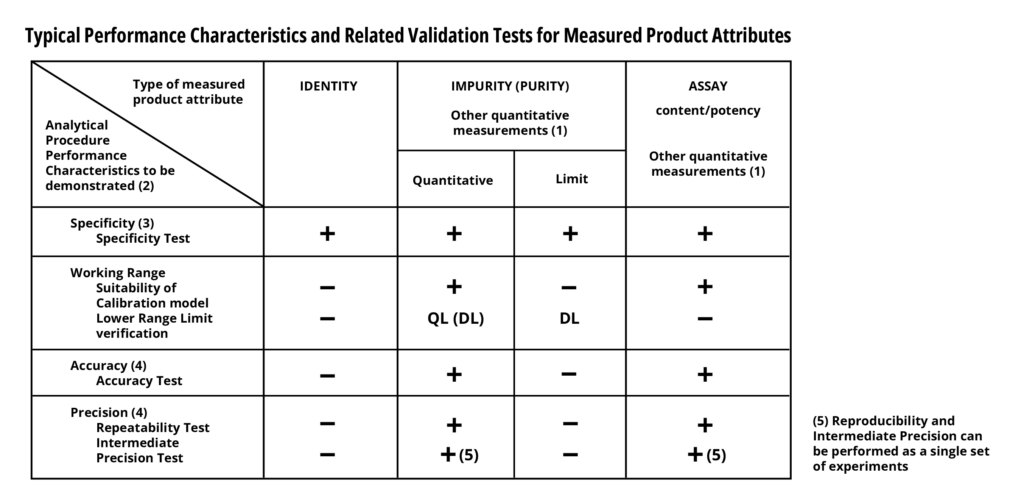
Along with this table were some specific suggestions about the number of samples/runs needed to sufficiently validate each characteristic. These suggestions were not particularly burdensome for most analytical methods, especially those which take hours to complete. However, cell-based and animal-based potency assays, which can take weeks to complete struggled with these recommendations.
In 2013, the United States Pharmacopeia (USP) published chapter <1033> on the Validation of Biological Assays. This guidance chapter proposed some statistical solutions to help alleviate the burdensome sample/run numbers suggested in ICHQ2(R1). At its heart, the USP chapter did not alter the broader approach of which performance characteristics needed to be validated. Nor did it alter the requirement for meeting pre-established acceptance validation criteria. This adherence to ICH Q2 principles may not be immediately obvious to new readers as, unfortunately, the USP chapter utilizes slightly different terminology from ICHQ2. Below is a guide to the different terms.
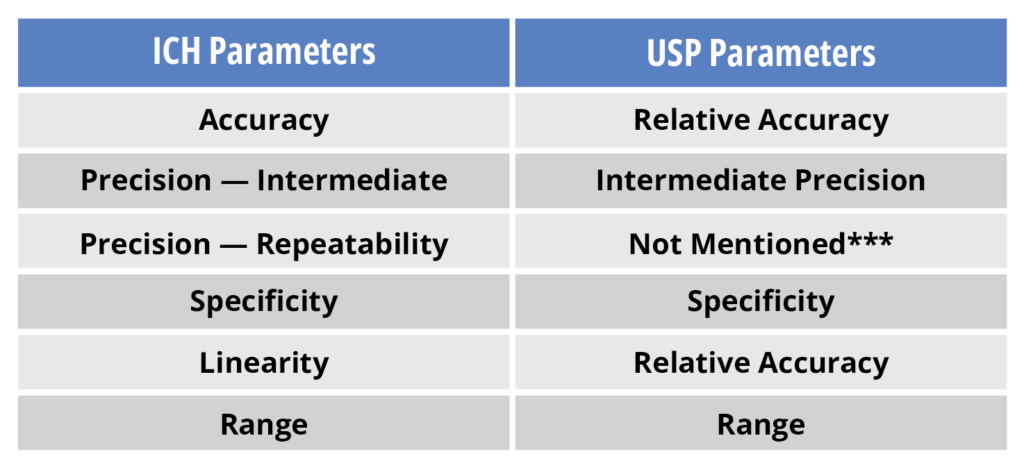
One of the novel concepts in the USP chapter was the introduction of more sophisticated tools to estimate precision. In particular, ICH Q2(R1) estimates assay precision at 3 to 5 different analyte levels within the assay while USP <1033> determines whether the precisions among these different concentrations are in fact similar, then combines these estimates to provide a single precision value for the method. Secondly ICHQ2(R1) requires that the assay be run in its entirety to calculate method precision. However, USP states that precision of the method can be determined using the simplest replication of the method.
This latter concept is quite complicated and a strict understanding of the meaning of the “simplest replication” is needed. The reportable value from a potency bioassay often requires a combination of assay runs. This is in direct contrast to many other analytical methods which require a single method run to give a reportable value. Below is an example of what is meant by simplest replication:
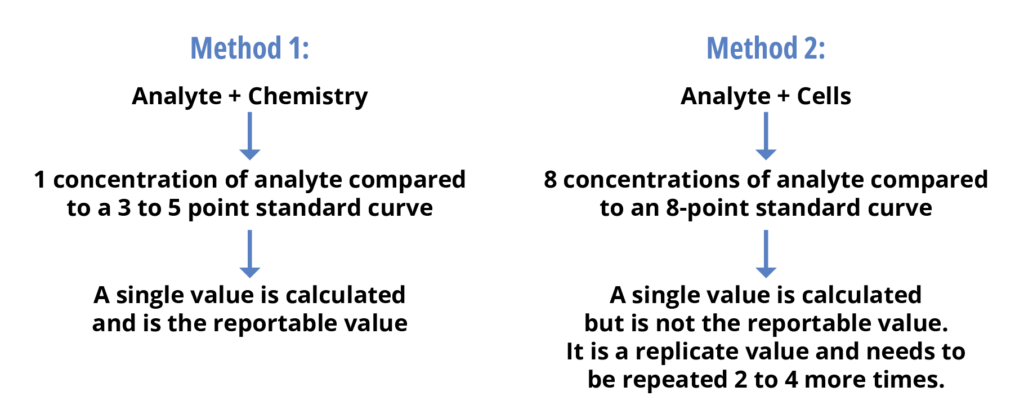
If one follows the ICH Q2(R1) concept that the entire method must be run to determine the precision, this means that to determine the method precision of method 1 above one would have to run the method 3 times. However, for method 2, the method would have to be run anywhere from 6 to 12 times. If each assay run takes 2 weeks, an ICH Q2(R1) precision validation would take anywhere from 12 to 24 weeks. Whereas a USP approach of using the single replicate would take 6 weeks.
The USP use of the “simplest assay replicate” relies on the well-known relationship of standard error (SE) of the mean to the number of replicates. Specifically, the standard deviation of a mean (Sm) is inversely proportional to the square root of the number of data (replicates), N. Specifically it is calculated by dividing the sample standard deviation by the square root of the sample size. This is mathematically written: Sm = S/√N. Using this relationship the precision validation criterion for a single replicate can be calculated and used rather than the method precision criterion. This single change to the ICH Q2(R1) standard approach can save companies months of validation time, without impacting the intent of the validation protocol. Another change suggested by the USP <1033> chapter is the combination of the precision estimates at the 3 or 5 different analyte levels. It is suggested that the upper, lower and mid analyte concentrations should be examined for significant differences in their precision. If in fact they are statistically similar USP recommends that these precision values can be pooled to yield a single precision estimate for the method. These two alternative approaches to assessing precision do not substantially differ from the intent of ICHQ2.
Accuracy is another characteristic which is assessed in a similar fashion in the two validation guidelines. Accuracy is essentially calculated as a spike and recovery experiment in both the ICH and USP validation guidelines. Accuracy is usually reported in one of two ways either as the mean percent recovery of a known added amount of analyte in the sample or as the difference between the mean and the accepted true value. These are mathematically written as follows.

ICH specifically mentions both of these approaches while USP only highlights the relative bias calculation. If you look at the data – they are essentially interchangeable values.
Total Analytical Error and its Role in Validation
For several years now, some of the statisticians in the analytical world have been pointing out that precision and accuracy both describe potential errors in the reportable value reported for any analytical test. Many maintain that evaluating precision and accuracy separately does not necessarily assess the combined impact of the two. They suggest that validation should instead include an assessment of the total analytical error (TAE). This opens two options for precision/accuracy assessments – two separate studies or a single combined study.
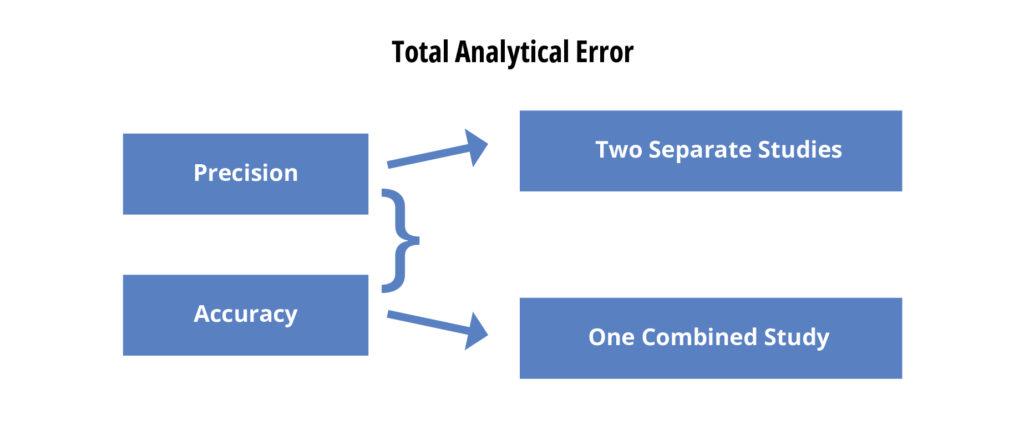
If one looks at a graphical representation of TAE (figure 5) one can see why this could become an important issue.
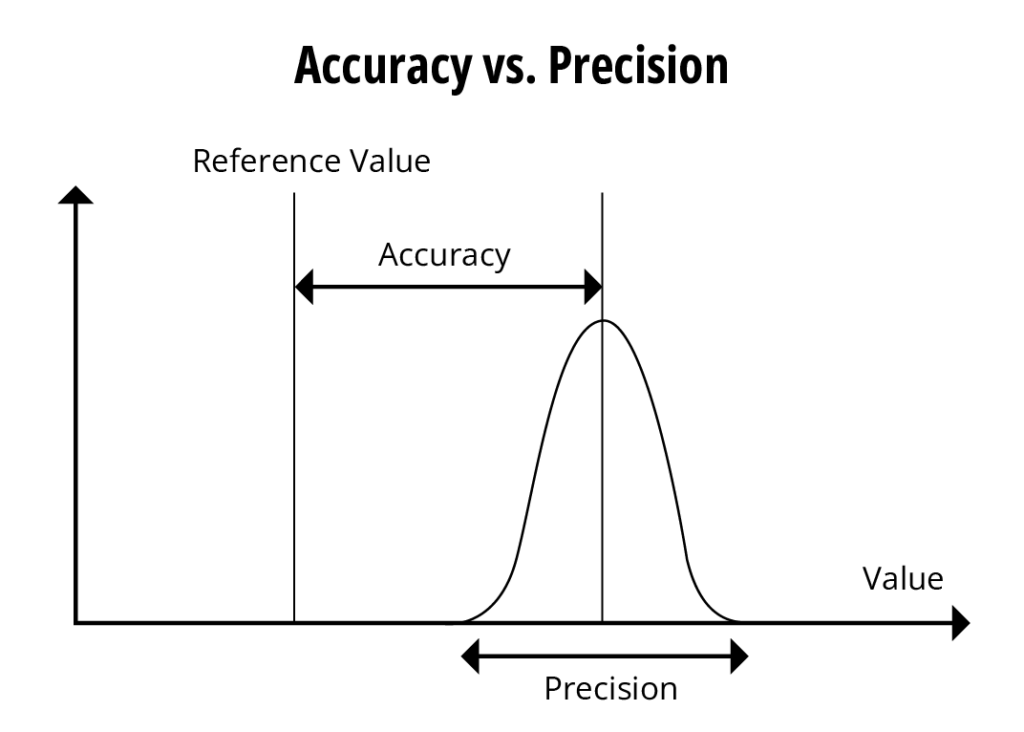
The total potential error of a reportable value must consider the accuracy of a measured value and its precision of the reading. Currently, firms establish conservative criteria for the two characteristics if they want to ensure appropriate measurements at the two ends of the analytical range.
Often times this can be viewed as TE = bias + 2 SD. But how specifically is it recommended that on measure and establish such a criterion?
The most recently approved ICH Q2(R2) (reference 1) states: “An alternative to separate evaluation of accuracy and precision is to consider their total impact by assessing against a combined performance criterion. The approach should be reflective of the individual criteria that would have been established for accuracy and precision.” While this is enabling it does not give any hints about how to implement such an approach.
The current rewrite of the USP <1033> (reference 2) also suggests that a TAE approach may be applicable for validation. (available for free from the USP PF here) This rewrite states “A TAE approach can be applied to the data at each level (and across levels) based on prediction intervals for the relative accuracy (%RB/100 + 1). This approach establishes whether combined RB and variability exceed the requirement for acceptable bioassay performance.“ An example of this calculation is included in the most recent available revision. It includes the bias ± 2 std. deviations but then adds in the manufacturing variability to the calculation.
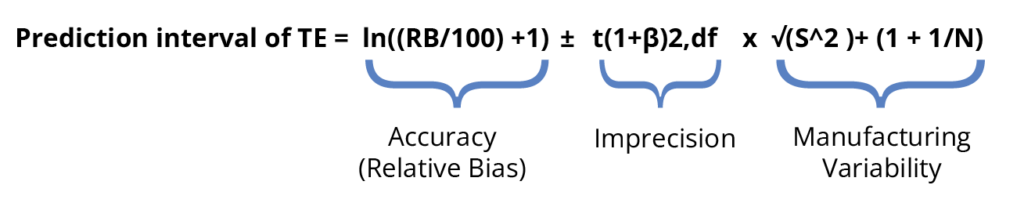
Currently, there are not enough numbers and hands-on calculations to aid a reader in learning how to implement a TAE approach for validation. However, there are predictions based upon numbers from methods which are precise but have very narrow specifications or methods which are less precise (reference 3) that a substantial portion of specification tolerance is taken up by the calculated tolerance interval for the TAE. This may result in methods failing validation. Fortunately neither guideline is currently recommending implementing only a TAE approach. So it will be up to the industry to investigate how to implement TAE into routine validation protocols and/or determining if the current separation of precision and accuracy criteria suffice to demonstrate a method’s suitability for use.
- ICH Q2(R2) implemented November 2023 available at https://database.ich.org/sites/default/files/ICH_Q2%28R2%29_Guideline_2023_1130.pdf
- USP Chapter <1033> Biological Assay Validation revision (comment period closed on 30 September 2024) https://online.uspnf.com/uspnf/document/2_GUID-952E8C3B-738B-40F8-A552-E14026AC78A9_102010201_en-US
- Bar, R. Total Analytical Error in Validation of Analytical Procedures of Pharmaceuticals. Pharmaceutical Technology2023 47 (5).

About The Author: Laureen Little
Dr. Laureen Little has over 30 years of biotechnology experience. She is the president of Biopharmaceutical Emerging Best Practices Association (BEBPA; www.bebpa.org) a non-profit organization she founded in in 2007 to promote scientific conferences for scientists working in the biopharmaceutical industry. BEBPA now hosts 3 to 4 conferences annually on such topics as potency bioassays, Host Cell Proteins, Immunogenicity assays, automation in analytical laboratories and the handling of reference materials. The organization has grown over the past decade and is now recognized as the venue for frank, open discussion and has resulted in vast improvements in technical approaches in various aspects of analytical development.
She is also a principal consultant with Quality Services, specializing in biological assay optimization and validation. She has been an instructor for FasTrain Courses (www.fastraincourses.com), for more than 20 years teaching various GXP courses as well as a popular potency assay course.
Laureen has worked with many firms developing, qualifying and validating biological potency assays for BLA submissions, Pre-Approval Supplements for commercial products and special amendments to support facility/manufacturing changes. The various products she has worked with include: monoclonal antibodies, rDNA products, peptide hormones, CBER regulated devices, gene therapy products, autologous cell therapies, toxins, cancer vaccines, viral vaccines, prophylactic vaccines, blood products, enzyme therapeutics, anti-angiogenic drugs, lipid-based therapies. The potency assays have included animal model systems, cell-based methods and binding assays.